Chapter 1 Introducing EMA
1.1 What is EMA?
Ecological Momentary Assessment (EMA) has many aliases. It is known as ‘Experience Sampling’ (R. Larson & Csikszentmihalyi, 1983), ‘Ambulatory Assessment’ (Ebner-Priemer & Trull, 2009) or ‘Ambulatory Self-reporting’ (Conner & Feldman Barrett, 2012), ‘Real-time Data Capturing’, the ‘Continuous Unified Electronic Diary Method’ (Ellis-Davies, Sakkalou, Fowler, Hilbrink, & Gattis, 2012), and as the ‘Intensive-longitudinal Study Design’ (Bolger & Laurenceau, 2013). The different terms stress different aspects of EMA research. All, however, refer to research methods that involve the repeated sampling of people’s current thoughts, emotions, behavior, physiological states, and context, in their natural environment, typically (but not necessarily) via electronic wearable devices (Shiffman et al., 2008).
1.1.1 Active versus Passive EMA
In EMA research, we distinguish two forms of data collection: 1) ‘Active EMA’, with which self-report data are collected, and 2) ‘Passive EMA’, with which observational data are collected. Active EMA requires participants to consciously provide information, for example by rating their current mood in response to a question that is prompted on their smartphone (see Figure 1.1). In passive EMA, information is collected through wearables or log files without active involvement of participants, for example on heart-rate, activity, smartphone use or engagement on social media (see Figure 1.2). Studies may combine active and passive EMA. A study into sleep patterns, for example, may involve both a self-report sleep diary and an accelerometer sensor (Van der Meijden et al., 2016).
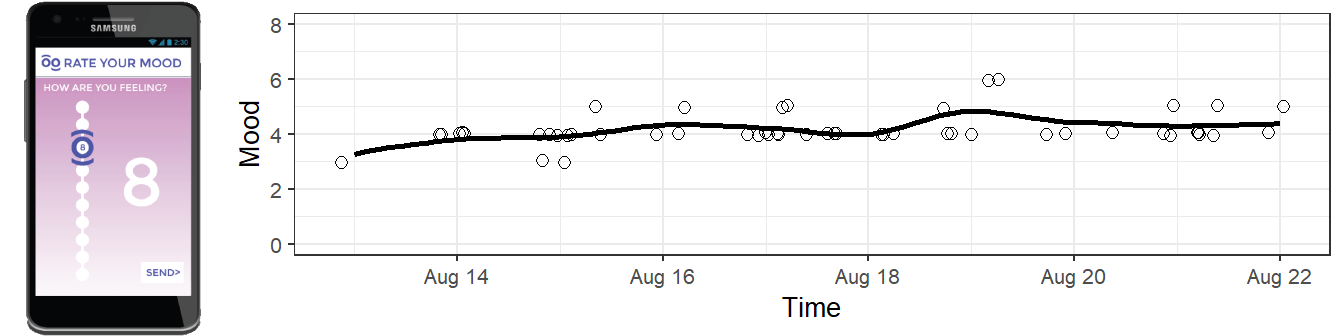
Figure 1.1: Active EMA: data are collected by prompting questions to participants, for instance by using an EMA app such as Moodbuster.
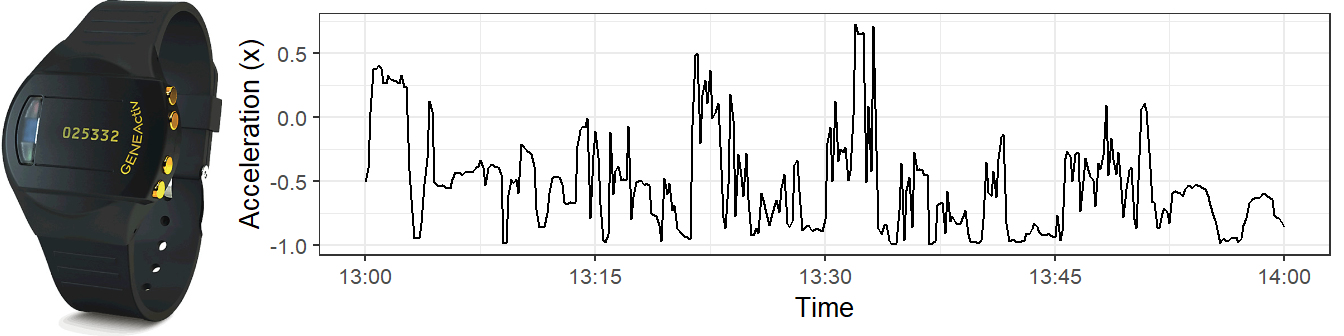
Figure 1.2: Passive EMA: data are collected automatically, for instance by a wearable device such as the GENEActiv accelerometer.
1.1.2 EMA Sampling
EMA is further typified by the way in which data sampling is triggered. EMA sampling may be triggered by a signal (signal-contingent sampling), an event (event-contingent sampling), or a combination of both (Conner & Lehman, 2012).
In signal-contingent sampling, participants respond to questions when they are prompted to do so by a signal (or ‘beeps’, as these signals are often called in the literature). In event-contingent sampling, study participants complete an assessment whenever a specific event occurs, such as a panic attack or alcohol consumption.
Signal-contingent sampling can follow a fixed or a random scheme. In a fixed scheme, participants are prompted at fixed time-points, for example at 9:30, 12:30, and 16:30. In a random scheme, prompts are sent at random time points, typically in pre-set intervals. For example, participants could be prompted to complete two assessments per day, one at a random time point between 10:00 and 14:00, and one at a random time point between 14:00 and 16:00. Using pre-set intervals ensures that participants do not receive several prompts within a limited time-frame (Piasecki, Hufford, Solhan, & Trull, 2007). In addition, it ensures that participants are not bothered by prompts at inappropriate times (e.g., participants may not appreciate prompts after 22:00 and before 7:30).
With ‘event-contingent sampling’, the sample rate is determined by the occurrence rate of the event. One way to implement this is to simply ask study participants to complete a questionnaire whenever the event occurs. When active EMA is combined with passive EMA, it may also be possible to trigger event-based prompts automatically based on changes in passive data, for example by triggering an EMA questionnaire automatically whenever a significant change in activity level is detected (Smyth & Stone, 2003).
1.2 Why EMA?
1.2.1 To Minimize Recall Bias
In clinical research, self-report questionnaires are often used to assess the presence and severity of symptoms in the recent past. While useful, these retrospective self-reports are not without drawbacks, since they tap into the memory of respondents, which can be distorted (Moore, Depp, Wetherell, & Lenze, 2016; Shiffman et al., 2008). EMA circumvents this recall bias, by asking participants to rate their current state, rather than asking them to reflect on past experiences.
1.2.2 To Maximize Ecological Validity
A key feature of EMA is the collection of data in real-world environments, as participants go about their daily activities, as opposed to data collection in controlled labs or research settings (Shiffman et al., 2008). Thus, EMA data can be expected to result in research findings that have better ecological validity and better generalization to the subject’s lived experience in real-world settings. Practical applications derived from EMA data are therefore expected to be more relevant to real-life situations.
1.2.3 To Advance Ideographic Research
In clinical research, a distinction is often made between ideographic and nomothetic methods (Allport, 1937). Idiographic methods are those that “aim to identify patterns of behavior within the person across a population of experiences or situations, and nomothetic methods[are] those that aim to identify patterns of behavior across a population of individuals, rather than for any given individual” (Conner, Tennen, Fleeson, & Barrett, 2009). The difference is important. As is increasingly recognized, group-level findings do not necessarily generalize to the individual members of the group, as shown by Figure 1.3 (Hamaker, 2012).
In contrast to more qualitative idiographic methods, such as interviews and N = 1 case studies, EMA allows for the collection of large amounts of quantitative data on the individual level. Thus, EMA offers a quantitative method for idiographic research, measuring characteristics of (unique) individuals across time and context (Shiffman et al., 2008). This allows for a better understanding of the factors that account for the variability within and between individuals.
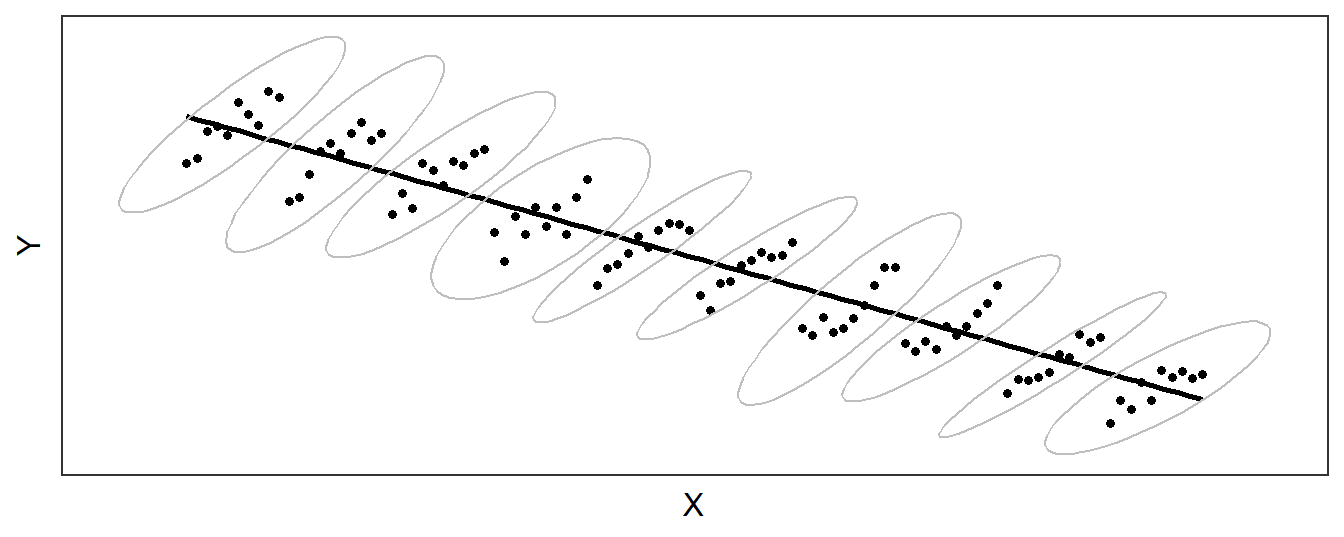
Figure 1.3: An illustration of how group-level and individual-level processes can differ dramatically: the relationship between x on y is negative in the group (as shown by the descending regression line), but positive for individuals (marked by ellipses).
1.2.4 To Understand the Dynamic Interplay between Symptoms
In the Network Theory of Psychopathology (Borsboom, 2017; Borsboom & Cramer, 2013), mental health disorders are conceptualized as networks of psychopathology symptoms, in which recurrent causal loops keep the network in a “disorder” state (e.g., sleeping problem -> fatigue -> rumination -> sleeping problem). Network Theory encourages the identification of patient-specific symptom networks, so that central symptoms can be targeted with personalized interventions, to break the self-sustaining loops. The identification of these networks requires repeated assessments of symptoms in real life (see, e.g., Bringmann, Lemmens, Huibers, Borsboom, & Tuerlinckx, 2015), a task for which EMA is particularly well suited.
1.2.5 To Enable EMI
EMA enables Ecological Momentary Interventions (EMI): interventions that are provided to people during their everyday lives, in real time, and in their natural settings (Heron & Smyth, 2010). If dynamic disease processes can be adequately monitored in everyday-life through EMA, it should also be possible to intervene when EMA data reflect clear changes in these processes, in a way that is maximally effective given what is known about the individual. A key term in the last sentence, however, is “adequately”. We should be aware of the “garbage-in/garbage-out” principle. Before EMI’s can be considered, the psychometric properties of EMA measures should be demonstrated first.
1.3 EMA Research Findings: A Birds-eye View
The use of EMA in mental health research might seem novel but this methodology has a long track record. Already in the 1980’s, early pioneers used electronic devices to elicit responses from study participants to tap into (mental) health processes in everyday life (see, e.g., M. Csikszentmihalyi & Larson, 2014). Recent years, however, have witnessed a large increase in EMA research. Rapid technological developments, a marked interest in the individual, and a wide recognition of the need to study health-related processes in real-life situations have all contributed to this.
EMA-based research in mental health has produced an impressive trove of findings that have supported and, sometimes, challenged existing theories on behavior. EMA data, whether collected as self-report or via wearable device/sensor, have diagnostic, monitoring, management, or intervention applications (Aung, Matthews, & Choudhury, 2017; Evenson, Goto, & Furberg, 2015; Patel, Park, Bonato, Chan, & Rodgers, 2012). Its feasibility for mental health research is evidenced by its use in observational studies and randomized controlled trials on a wide range of topics and populations. Below, we present a non-exhaustive summary of systematic reviews and meta-analyses of EMA research.
1.3.1 Active EMA
Mood disorders have been well-studied using active EMA methods (Wenze & Miller, 2010), with several reviews outlining recent findings in depression (C. Burton et al., 2013; Telford, McCarthy-Jones, Corcoran, & Rowse, 2012; Wichers et al., 2011), anxiety disorders (Walz, Nauta, & Aan het Rot, 2014), and depression/bipolar disorder (Aan het Rot, Hogenelst, & Schoevers, 2012). The potential of EMA for use among young populations showed promising results (Dubad, Winsper, Meyer, Livanou, & Marwaha, 2018). Innovations in mobile devices have improved the feasibility and popularity of ecological momentary interventions (EMIs) for anxiety and depression (Schueller, Aguilera, & Mohr, 2017). A systematic review and meta-analysis of EMIs reported small to medium effects on mental health (Versluis, Verkuil, Spinhoven, Van der Ploeg, & Brosschot, 2016).
EMA appears to be particularly well-suited to examine the role of emotions in the development and maintenance of obesity and eating disorders (Engel et al., 2016). Meta-analytic results suggest that negative affect, rather than hunger, is associated with binge eating among individuals with eating disorders (Haedt-Matt & Keel, 2011; Haedt-Matt, Zalta, Forbush, & Keel, 2012). EMA methods have also significantly contributed to the understanding of the processes that drive substance use, cessation, and relapse, often in contrast with theory-driven studies largely derived from global reports collected through retrospective questionnaires (Shiffman, 2009; Swendsen, 2016).
1.3.2 Passive EMA
Objective EMA data collected passively through bio-sensors, smart devices, or context/environmental (e.g. location) has been shown to be a feasible and promising method for the longitudinal monitoring of individuals with affective disorders (Dogan, Sander, Wagner, Hegerl, & Kohls, 2017; Kirchner & Shiffman, 2016). The potential of passive sensing via smartphone for mental health research are outlined in two reviews, encompassing the assessment of health and well-being (Cornet & Holden, 2018), and the measuring, understanding, and intervening in mental illness and maintaining mental health (Aung et al., 2017). A systematic review and meta-analysis on actigraphy reported diurnal variations in activity levels among individuals with depression (C. Burton et al., 2013). Compared with traditional self-reports, passive sensing was reported to be less intrusive and to result in more accurate data, while providing options for continuous monitoring and feedback.
1.3.3 Limitations
While available reviews suggest that EMA is a feasible research method that has the potential to make significant contributions to mental health research, one significant drawback of EMA highlighted in several reviews is the lack of high-quality studies. Other general limitations considered include:
Unclear generalizability of research findings due to selective samples (C. Burton et al., 2013), and small sample sizes (Dogan et al., 2017)
Unclear practice effects (Telford et al., 2012), and issues related to reactivity (Shiffman, 2009)
Issues related to the feasibility and tolerability of prolonged and intense data periods of data collection (Wichers et al., 2011)
Issues of privacy, ethics and informed consent (Cornet & Holden, 2018)
Changing responsibilities of researchers and clinicians in EMA studies, e.g. in suicide ideation research (Wenze & Miller, 2010)
Unresolved methodological issues (Dubad et al., 2018)
Topic / Author (Year) | Summary |
---|---|
Anxiety disorders | |
Walz et al. (2014) | Provides insights to the temporal variability of symptoms, and associations between daily affect, behaviors, and situational cues. Discusses the combination of EMA and ambulatory assessment of physiological variables in treatment evaluations. |
Schueller et al. (2017) | Provides an overview of the distinction of EMIs from other types of treatment. Also discusses the considerations of conducting EMI research, such as design, deployment, and evaluation. |
Eating disorders | |
Engel et al. (2016) | An overview of studies on eating disorders, obesity, and bariatric surgery using EMA. |
Mood disorders | |
Aan het Rot et al. (2012) | Provides an overview of EMA studies on correlates of mood, treatment effects, residual symptoms of remitted patients, pediatric populations, MDD/BD specificity, and links with neuroscience. |
Aung et al. (2017) | Provides a conceptual review of passive sensing techniques for measuring, understanding, and treatment of mental illness. |
C. Burton et al. (2013) | Focuses on diurnal variations in activity levels among depressed individuals. |
Telford et al. (2012) | Identifies six themes of EMA research in MDD: methodology, positive and negative affect, cortisol secretion, antidepressant treatment, work performance, and genetic risk factors. |
Wenze & Miller (2010) | Provides an overview of EMA in mood disorder research comprising techniques used, types of population assessed, types of research questions, and a discussion of the potential of EMA in treatment settings. |
Wichers et al. (2011) | Provides an overview for the potential clinical application of EMA in the diagnostic and treatment of MDD. |
Versluis et al. (2016) | Provides an overview of interventions (EMI) addressing anxiety, depression, and perceived stress on positive psychological outcomes. |
Dubad et al. (2018) | Provides an overview of the feasibility and clinical impact of mood-monitoring applications targeting young populations (10-24 years old). |
Substance-related disorders | |
Shiffman (2009) | Review of processes that drive substance use, cessation, and relapse, sometimes in contrast with theory-driven studies that are largely derived from global reports collected through questionnaires. |
Swendsen (2016) | Conceptual review of the use of mobile technologies for research on addiction and its treatment. |
Mental health/ Well-being | |
Cornet & Holden (2018) | Outlines the potential and challenges of passive sensing to detect status change and behavior change following feedback on behavior. |
Kirchner & Shiffman (2016) | Provides an overview of ‘geographically explicit momentary assessment’ (GEMA) research to enrich EMA research in mental health and well-being. |
Dogan et al. (2017) | Provides an overview of studies that combine subjective ratings with objective EMA-data collected using smartphone-based systems. |
1.4 What is in this Manual?
This manual comprises six parts, in which various aspects of EMA research are discussed.
Chapter 2, the second chapter of Part I, introduces R, the statistical program that is an indispensable tool for the EMA researcher.
Part II focuses on EMA study design (Chapter 3), and EMA data management (Chapter 4).
Part III details the momentary assessment of two specific outcomes: Mood (Chapter 5) and Activity (Chapter 6).
Part IV discusses EMA data analysis techniques: Feature Extraction (Chapter 7), and Mixed Modeling (Chapter 8).
In part V, the application of the preceding material is illustrated in a case study, in which EMA is used to detect early warnings signs of depression (Chapter 9).
Part VI provides three catalogues of EMA resources. Chapter 10 lists EMA research groups within APH, chapter 11 lists EMA instruments that were found to be in use among APH researchers, and Chapter 12 summarizes useful R extensions (packages) for EMA data analysis.
References
Larson, R., & Csikszentmihalyi, M. (1983). The experience sampling method. In H. T. Reis (Ed.), Naturalistic approaches to studying social interaction (pp. 41–56). San Francisco: Jossey-Bass Inc Pub.
Ebner-Priemer, U. W., & Trull, T. J. (2009). Ecological momentary assessment of mood disorders and mood dysregulation. Psychological Assessment, 21(4), 463–475. https://doi.org/10.1037/a0017075
Conner, T. S., & Feldman Barrett, L. (2012). Trends in ambulatory self-report: The role of momentary experience in psychosomatic medicine. Psychosomatic Medicine, 74(4), 327–337. https://doi.org/10.1097/PSY.0b013e3182546f18
Ellis-Davies, K., Sakkalou, E., Fowler, N. C., Hilbrink, E. E., & Gattis, M. (2012). CUE: The continuous unified electronic diary method. Behavior Research Methods, 44(4), 1063–1078. https://doi.org/10.3758/s13428-012-0205-1
Bolger, N., & Laurenceau, J.-p. (2013). Introduction to intensive methods. In Intensive longitudinal methods: An introduction to diary and experience sampling research. New York: Guilford Press.
Shiffman, S., Stone, A. A., Hufford, M. R., Rev, A., Psychol, C., Shiffman, S., … Hufford, M. R. (2008). Ecological Momentary Assessment. Annual Review of Clinical Psychology, 4(1), 1–32. https://doi.org/10.1146/annurev.clinpsy.3.022806.091415
Van der Meijden, W. P., Van Someren, J. L., Te Lindert, B. H., Bruijel, J., Van Oosterhout, F., Coppens, J. E., … Van Someren, E. (2016). Individual differences in sleep timing relate to melanopsin-based phototransduction in healthy adolescents and young adults. Sleep, 39(6), 1305–1310. https://doi.org/10.5665/sleep.5858
Conner, T. S., & Lehman, B. J. (2012). Getting started: Launching a study in daily life. In M. R. Mehl & T. S. Conner (Eds.), Handbook of research methods for studying daily life. (pp. 89–107). New York, NY, US.: Guilford Press.
Piasecki, T. M., Hufford, M. R., Solhan, M., & Trull, T. J. (2007). Assessing clients in their natural environments with electronic diaries: Rationale, benefits, limitations, and barriers. Psychological Assessment, 19(1), 25–43. https://doi.org/10.1037/1040-3590.19.1.25
Smyth, J. M., & Stone, A. A. (2003). Ecological momentary assessment research in behavioral medicine. Journal of Happiness Studies, 4(1), 35–52. https://doi.org/10.1023/A:1023657221954
Moore, R. C., Depp, C. A., Wetherell, J. L., & Lenze, E. J. (2016). Ecological momentary assessment versus standard assessment instruments for measuring mindfulness, depressed mood, and anxiety among older adults. Journal of Psychiatric Research, 75, 116–123. https://doi.org/10.1016/j.jpsychires.2016.01.011
Allport, G. W. (1937). Personality: A psychological interpretation. Oxford, England: Holt; Company.
Conner, T. S., Tennen, H., Fleeson, W., & Barrett, L. F. (2009). Experience sampling methods: A modern idiographic approach to personality research. Social and Personality Psychology Compass, 3(3), 292–313. https://doi.org/10.1111/j.1751-9004.2009.00170.x]
Hamaker, E. (2012). Why researchers should think “within-person”: A paradigmatic rationale. Handbook of Research Methods for Studying Daily Life, 43–61. https://doi.org/10.1007/978-3-531-93094-7
Borsboom, D. (2017). A network theory of mental disorders. World Psychiatry. https://doi.org/10.1002/wps.20375
Borsboom, D., & Cramer, A. O. J. (2013). Network analysis: An integrative approach to the structure of psychopathology. Annual Review of Clinical Psychology, 9(1), 91–121. https://doi.org/doi:10.1146/annurev-clinpsy-050212-185608
Bringmann, L. F., Lemmens, L. H., Huibers, M. J., Borsboom, D., & Tuerlinckx, F. (2015). Revealing the dynamic network structure of the Beck Depression Inventory-II. Psychological Medicine. https://doi.org/10.1017/S0033291714001809
Heron, K. E., & Smyth, J. M. (2010). Ecological momentary interventions: Incorporating mobile technology into psychosocial and health behaviour treatments. British Journal of Health Psychology, 15(1), 1–39. https://doi.org/10.1348/135910709X466063
Csikszentmihalyi, M., & Larson, R. (2014). Validity and reliability of the experience-sampling method. In Flow and the foundations of positive psychology: The collected works of mihaly csikszentmihalyi (pp. 35–54). Dordrecht: Springer Netherlands. https://doi.org/10.1007/978-94-017-9088-8_3
Aung, M. H., Matthews, M., & Choudhury, T. (2017). Sensing behavioral symptoms of mental health and delivering personalized interventions using mobile technologies. Depression and Anxiety, 34(7), 603–609. https://doi.org/10.1002/da.22646
Evenson, K. R., Goto, M. M., & Furberg, R. D. (2015). Systematic review of the validity and reliability of consumer-wearable activity trackers. International Journal of Behavioral Nutrition and Physical Activity, 12(1), 159. https://doi.org/10.1186/s12966-015-0314-1
Patel, S., Park, H., Bonato, P., Chan, L., & Rodgers, M. (2012). A review of wearable sensors and systems with application in rehabilitation. Journal of Neuroengineering and Rehabilitation, 9(1), 21. https://doi.org/10.1186/1743-0003-9-21
Wenze, S. J., & Miller, I. W. (2010). Use of ecological momentary assessment in mood disorders research. Clinical Psychology Review, 30(6), 794–804. https://doi.org/10.1016/j.cpr.2010.06.007
Burton, C., McKinstry, B., Tătar, A. S., Serrano-Blanco, A., Pagliari, C., & Wolters, M. (2013). Activity monitoring in patients with depression: A systematic review. Journal of Affective Disorders, 145(1), 21–28. https://doi.org/10.1016/j.jad.2012.07.001
Telford, C., McCarthy-Jones, S., Corcoran, R., & Rowse, G. (2012). Experience sampling methodology studies of depression: The state of the art. Psychological Medicine, 42(6), 1119–1129. https://doi.org/10.1017/S0033291711002200
Wichers, M., Simons, C. J. P., Kramer, I. M. A., Hartmann, J. A., Lothmann, C., Myin-Germeys, I., … Van Os, J. (2011). Momentary assessment technology as a tool to help patients with depression help themselves. Acta Psychiatrica Scandinavica, 124(4), 262–272. https://doi.org/10.1111/j.1600-0447.2011.01749.x
Walz, L. C., Nauta, M. H., & Aan het Rot, M. (2014). Experience sampling and ecological momentary assessment for studying the daily lives of patients with anxiety disorders: A systematic review. Journal of Anxiety Disorders, 28(8), 925–937. https://doi.org/10.1016/j.janxdis.2014.09.022
Aan het Rot, M., Hogenelst, K., & Schoevers, R. A. (2012). Mood disorders in everyday life: A systematic review of experience sampling and ecological momentary assessment studies. Clinical Psychology Review, 32(6), 510–523. https://doi.org/10.1016/j.cpr.2012.05.007
Dubad, M., Winsper, C., Meyer, C., Livanou, M., & Marwaha, S. (2018). A systematic review of the psychometric properties, usability and clinical impacts of mobile mood-monitoring applications in young people. Psychological Medicine, 48(2), 208–228. https://doi.org/10.1017/S0033291717001659
Schueller, S. M., Aguilera, A., & Mohr, D. C. (2017). Ecological momentary interventions for depression and anxiety. Depression and Anxiety, 34(6), 540–545. https://doi.org/10.1002/da.22649
Versluis, A., Verkuil, B., Spinhoven, P., Van der Ploeg, M. M., & Brosschot, J. F. (2016). Changing mental health and positive psychological well-being using ecological momentary interventions: A systematic review and meta-analysis. Journal of Medical Internet Research, 18(6). https://doi.org/10.2196/jmir.5642
Engel, S. G., Crosby, R. D., Thomas, G., Bond, D., Lavender, J. M., Mason, T., … Wonderlich, S. A. (2016). Ecological momentary assessment in eating disorder and obesity research: A review of the recent literature. https://doi.org/10.1007/s11920-016-0672-7
Haedt-Matt, A. A., & Keel, P. K. (2011). Hunger and binge eating: A meta-analysis of studies using ecological momentary assessment. International Journal of Eating Disorders, 44(7), 573–578. https://doi.org/10.1002/eat.20868
Haedt-Matt, A. A., Zalta, A. K., Forbush, K. T., & Keel, P. K. (2012). Experimental evidence that changes in mood cause changes in body dissatisfaction among undergraduate women. Body Image, 9(2), 216–220. https://doi.org/10.1016/j.bodyim.2011.11.004
Shiffman, S. (2009). Ecological momentary assessment (EMA) in studies of substance use. Psychological Assessment, 21(4), 486–497. https://doi.org/10.1037/a0017074
Swendsen, J. (2016). Contributions of mobile technologies to addiction research. Dialogues in Clinical Neuroscience, 18(2), 213. Retrieved from https://www.ncbi.nlm.nih.gov/pmc/articles/PMC4969708/
Dogan, E., Sander, C., Wagner, X., Hegerl, U., & Kohls, E. (2017). Smartphone-based monitoring of objective and subjective data in affective disorders: Where are we and where are we going? Systematic review. Journal of Medical Internet Research, 19(7). https://doi.org/10.2196/jmir.7006
Kirchner, T. R., & Shiffman, S. (2016). Spatio-temporal determinants of mental health and well-being: Advances in geographically-explicit ecological momentary assessment (gema). Social Psychiatry and Psychiatric Epidemiology, 51(9), 1211–1223. https://doi.org/10.1007/s00127-016-1277-5
Cornet, V. P., & Holden, R. J. (2018). Systematic review of smartphone-based passive sensing for health and wellbeing. Journal of Biomedical Informatics, 17(1), 120–132. https://doi.org/10.1016/j.jbi.2017.12.008